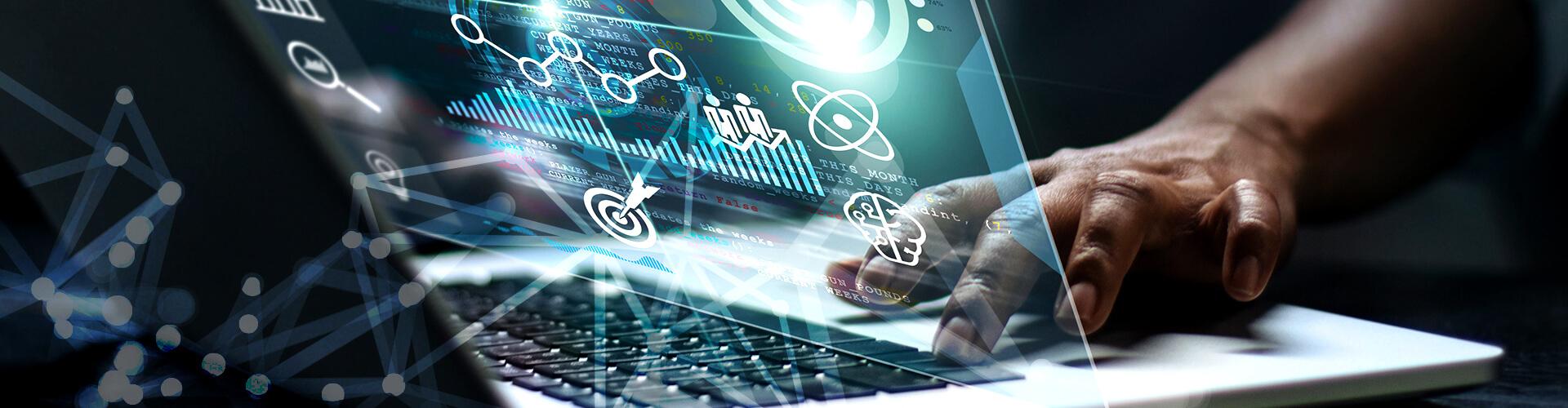
Micro-Credential in Advanced Data Science
Program Overview
This page contains information and guidelines for students interested in pursuing the Micro-credential in Advanced Data Science from the Hewlett Packard Enterprise Data Science Institute (HPE DSI).
The purpose of the Micro-credential in Advanced Data Science is to recognize the expertise gained by students during their studies in the areas of:
Core Courses
- 211 Data Analysis in R
- 311 Introduction to Machine Learning
- 312 Introduction to Deep Learning
Elective Courses
- 221 Data Science & Scientific Computing using Julia
- 271 Data Analysis & Visualization using Excel & PowerBI
Cost
- Free for active University of Houston System (UHS) students, staff or faculty
- $250/course badge for non-UH individuals
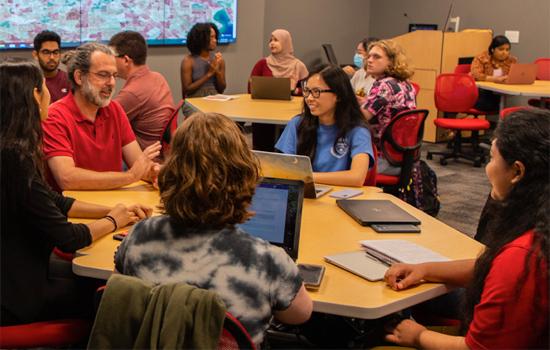
How does it work?
4 Badges = 1 Micro-credential
Register and complete each of the four courses below and the HPE DSI will automatically award you the Micro-credential in Advanced Data Science.
The Badge for the Micro-credential will be awarded at the end of each semester.
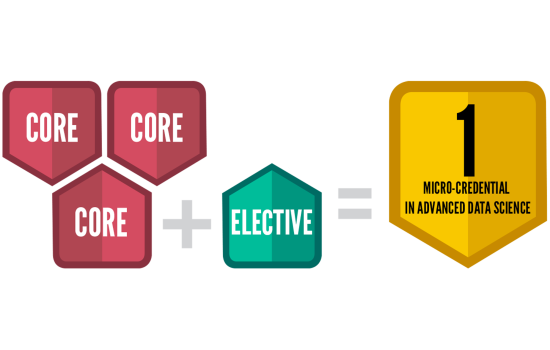
Core Course Descriptions
Please note, to receive the Micro-credential badge, make sure to complete the courses listed below.
These courses will neither affect your GPA nor appear in your transcripts.
The description for each course can be found below:
This course will teach you a basic understanding of how to program in R. You will learn how to use the R Studio software necessary for a statistical programming environment. The course covers reading data in R, accessing R packages, writing R functions, debugging and commenting on R code. We will introduce visualization concepts in R, and you will also learn how to run R code on the HPE DSI HPC (High Performance Computing) clusters.
Introduction to Machine Learning
Machine learning is the science of developing statistical methods that quantify relationships within data. This branch of mathematics/computer science has seen explosive growth over the past decade as our ability to store and process digital data has dramatically increased. This course will enable students to: obtain an overview of the literature in learning-based methods and applications, understand a variety of machine learning techniques for classification, regression and prediction and obtain the ability to implement and experiment with a wide range of machine learning algorithms in Python. Also covered will be Unsupervised and Supervised learning and clustering concepts, Dimensional Reduction, kernels, and kernel-based classifiers such as SVM, and Deep Learning algorithms. The course will enable participants to understand and implement learning-based methods for the classification of images, signals and features.
Please Note: This course requires familiarity with some basic concepts which are present in most programming languages: primitive and non-primitive data structures and operators, conditional and repeated execution, and working with libraries/modules. All programming work in this course will be done using Python, a beginner-friendly language.
Deep learning refers to AI/ML techniques that utilize multilayer (deep) artificial neural networks. This branch of data science has seen exponential improvements in performance as our ability to collect, store, and process digital data has dramatically increased. Prediction, classification, regression, and identification of semi/unstructured data are areas where deep learning techniques exhibit a significant comparative advantage.
Please Note: This course requires familiarity with some basic concepts which are present in most programming languages: primitive and non-primitive data structures and operators, conditional and repeated execution, and working with libraries/modules. All programming work in this course will be done using Python, a beginner-friendly language.
Elective Courses
Please note, to receive the Micro-credential badge, make sure to complete the courses listed below.
These courses will neither affect your GPA nor appear in your transcripts.
The description for each course can be found below:
Data Science and Scientific Computing using Julia
This course is an introduction to the Julia programming language. Julia is the fastest modern open-source language for data science, machine learning and scientific computing. Julia provides the functionality, ease-of-use and intuitive syntax of R, Python, Matlab, SAS, or Stata combined with the speed, capacity, and performance of C, C++, or Java. Julia also provides parallel and distributed computing capabilities and unlimited scalability with minimal effort.
Data Analysis & Visualization in Excel & PowerBI
This hands-on course introduces the key concepts for business data analytics using both MS Excel and Power BI, serving as an analytics literacy course to help students gain essential knowledge in business data analytics to enhance firm competitiveness. Concepts introduced in this course aim to develop an understanding of the several types of business data, data modeling techniques, various analytical approaches, and the application of these approaches to solve business problems using platform-agnostic tools in MS Excel and Power BI. The practices cover both basic and advanced functions of MS Excel, including PivotTable, PivotChart, and Adds-in, as well as key features of Power BI such as data connections, report designing, and interactive report creation. No textbooks are required.
Registration
UH Students
- Login to Moodle to sign up for each course (access this link via campus WIFI or the UH VPN).
Non-UH/Alumni
- Complete the payment of the course fees ($250.00 per course) at least three (3) weeks before course commencement. The URL and payment links are available on the course page.
- Inform the HPE Data Science Institute by email of your interest in taking the course at least three (3) weeks before the course start date. This will allow adequate time for processing of your course access credentials before the first-class meeting.
**You may email us at contact@hpedsi.uh.edu for additional information.
Retroactive Badges
Please email the HPE Data Science Institute if you have completed any of the four HPE DSI courses listed on this page on or after the Spring 2019 semester.
Micro-Credential Badges
All the students who have successfully completed the Micro-credential in Advanced Data Science program are encouraged to fill out the badge petition form.
Micro-credential in Advanced Data Science Application Form
**Please note: The form can only be filled by students who have received the badges for all the courses (as listed on this web page).
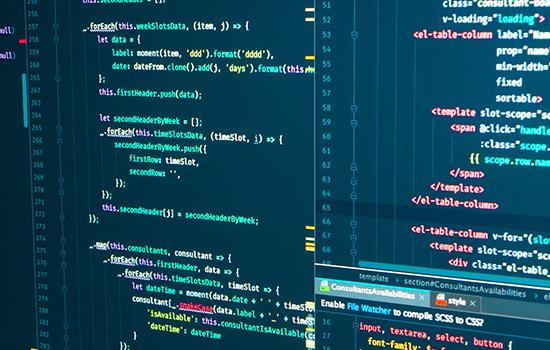
Badgr Instructions
Link your HPE DSI Badges to a Badgr account and share them on online platform (e.g., LinkedIn).
Create a Badgr account at badgr.io if you don’t already have one.
- Enter the sign in credentials for your Badgr account in the Moodle Backpack page to request to connect.
- If successful, the backpack status will change to “Verification pending”.
- Check the email account you registered with Badgr for a verification email from HPE DSI.
- Click on the verification link in the email to confirm and activate the connection of your Moodle backpack to your Badgr backpack.
- If successful, the link will take you to your Moodle Backpack page and the status will change to "connected".
- Go to the “manage my badges” page, where you will see a list of all your earned HPE DSI Badges.
- Click on the specific HPE DSI Badge you want to share; the link will take you to a details page about the Badge.
- Click on the "Add to Backpack" button.
- If successful, you will be taken back to the “manage my badges” page, which will now display an “Added badge to backpack” notice. You will also receive an email from Badgr noting that you have earned this Badge.
- Sign in to your badgr.io account.
- Select the Badge you want to share from the list.
- Click on “Share” to generate a URL or link it to social platforms; Click on "Download" to save it on your computer device.