Accelerated Materials Design Driven by Machine Learning
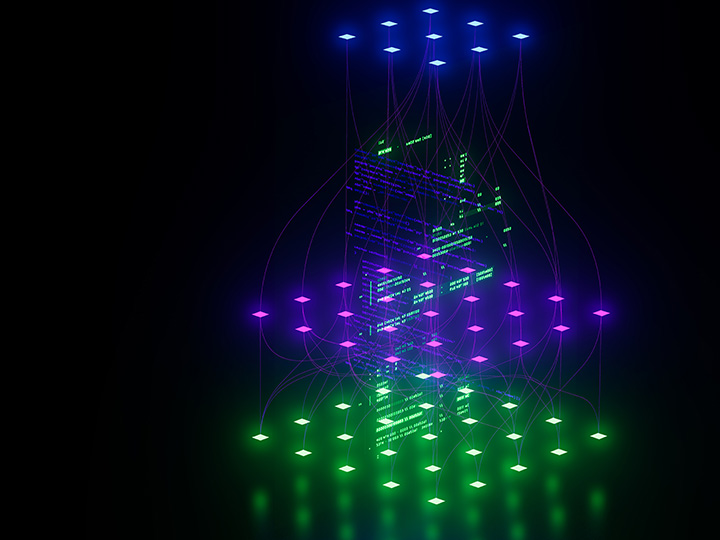
On March 21, 2024, the University of Houston Hewlett Packard Enterprise Data Science Institute hosted an online research lecture titled "Accelerated Materials Design Driven by Machine Learning" featuring Mingjian Wen, an assistant professor of Chemical and Biomolecular Engineering at UH's Cullen College of Engineering.
Wen’s lecture highlighted two main challenges in creating reliable artificial intelligence models for materials science: uncertainty quantification for neural network models and the incorporation of prior physical knowledge and constraints into such models.
Wen's research employs graph neural networks to model the elastic properties of materials. From the predicted elastic tensor, one can obtain various mechanical properties, such as bulk modulus (resistance to volume change) and shear modulus (resistance to sliding deformation) across different crystal systems. This capability is particularly valuable because many materials exhibit anisotropy, behaving differently depending on the direction of applied force.
Wen stressed the importance of incorporating physical constraints and uncertainty quantification into these models. He explained how a dropout technique can be used to assess the reliability of predictions, especially when extrapolating beyond the training data. This approach allows researchers to identify when a model might be unreliable for certain materials or conditions.
The lecture highlighted practical applications of these AI-driven methods. Wen demonstrated how the models could accelerate materials innovation by rapidly screening thousands of potential candidates and identifying those with exceptional properties such as strength. Wen emphasized that the true power of these methods lies in combining traditional scientific knowledge with machine learning techniques. This synergy between human expertise and AI tools has the potential to revolutionize materials design and discovery across various industries, creating more effective and physically-justified models for materials science.
Mingjian Wen, Ph.D is a Presidential Frontier Faculty Fellow at the University of Houston. His current work focuses on developing data-driven approaches for computational understanding and design of energy materials and contributing to open cyberinfrastructure for materials modeling. Prior to UH, Wen worked as a postdoctoral scholar on the Materials Project at Lawrence Berkeley National Lab. Wen's lecture is part of an ongoing series hosted by the HPE Data Science Institute, aimed at showcasing cutting-edge research that leverages high-performance computing resources for scientific discovery.