Data Science and Machine Learning: The Weaker The Better
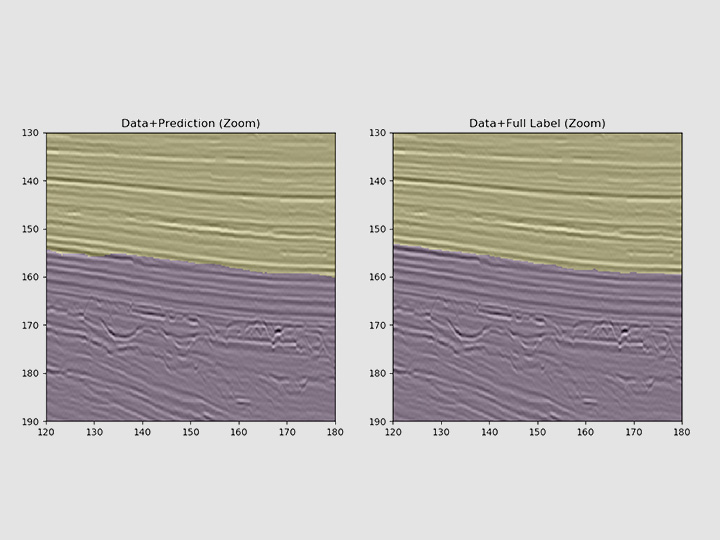
In partnership with the HPE Data Science Institute and Geophysical Society of Houston, Bas Peters, visiting assistant professor at Emory University, delivered a presentation about training neural networks for seismic interpretation using image-level information.
Regarding supervised learning, Peters emphasized the distinctive settings to train neural networks for semantic segmentation. In seismic and geophysical data, the labels often come from ground-truth measurements and are sparsely available. Sometimes, manual annotation can hinder your research due to it being time-consuming, expensive, and occasionally unreliable.
To develop high-quality automated seismic interpretation, a computer vision system must learn without seeing fully annotated examples. There are different levels of supervision, such as full, image-level and point-level. Peters presented a constrained optimization approach that injects quantitative image-level knowledge information about the general geological structure into the learning problem to supplement sparse annotation. This technique enables training neural networks with fewer annotations.
An approach to dealing with sparse labels is by training the patch within the label and classifying it by central pixels. Researchers can utilize different formulations to configure partial annotations and constraints to produce effective segmentation and train their network.