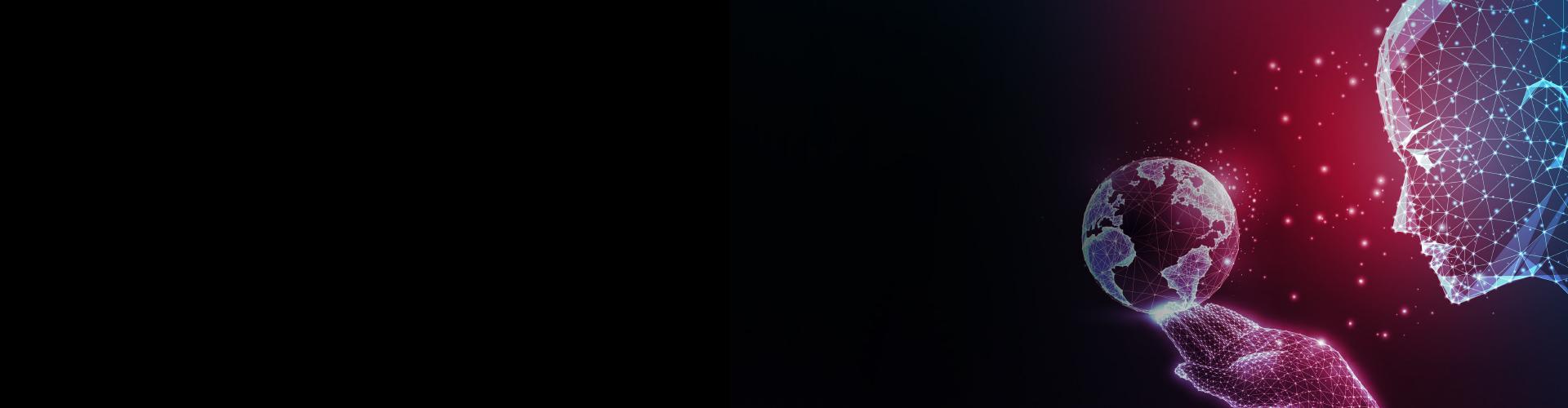
AI and Data Science Showcase
Share Your Projects
Hosted by the Department of Computer Science and the HPE Data Science Institute, the AI and Data Science Showcase recognizes outstanding young researchers who have shown a commitment to the fields of computing and data science.
All team members must be undergraduate students or graduate students, currently enrolled within the University of Houston System. Students from all academic disciplines are welcome. Cross-disciplinary teams and projects employing artificial intelligence, machine learning, deep learning, and neural networks are especially encouraged.
Applications for the May showcase are now closed.
- Open to undergraduate or graduate students (master's or doctoral) currently enrolled at the University of Houston.
- Teams can be cross-disciplinary, encouraging members from diverse fields of study to participate.
- Each team must consist of a minimum of 2 and a maximum of 5 participants.
Categories:
Undergraduate Category: Teams will consist of undergraduate students from any discipline. Cross-disciplinary teams are encouraged to bring diverse perspectives.
Graduate Category: Teams will consist of graduate students (master's or doctoral) from any discipline. Cross-disciplinary teams are encouraged to foster diverse skill sets and knowledge.
Original Work and Data Usage:
- The work must be completed between June 2024 until now and should not have been submitted previously as a class project or to another competition.
- Pre-existing code or datasets are allowed, provided they are publicly available and appropriately credited.
- If external datasets are used, they must be publicly accessible, and participants must adhere to the terms of use.
Tools & Software:
Participants can use any legally licensed open-source tools, libraries, or frameworks to create their projects, such as Python, R, etc.
Participants must submit their projects using the designated AI-Showcase Poster Submission Form.
Documents to be submitted:
- Poster (using the provided template)
- PowerPoint Presentation (based on the poster)
Important Notes:
- All submissions must be uploaded as either .pdf or .pptx files.
- Posters must use the poster template provided here: Poster Template
- Prepare the PowerPoint of your poster and submit it.
- Projects must be submitted by the specified deadline. Late submissions will not be considered.
- Code and data files are not required. Submissions should include only the poster and a presentation that reproduces the poster.
- Please include your designated Team number on each slide and poster. You will find the unique team number in the acknowledgment email sent after registering for the competition.
Intellectual Property:
- Unless stated otherwise, participants retain the rights to their projects. However, the organizing body may request permission to highlight or promote projects for marketing purposes
Withdrawal Policy:
- If a participant or team needs to withdraw from the competition, they must notify the organizing committee by email at least one week before the final presentation date.
Mentorship:
Participants are encouraged to seek guidance from mentors. However, mentors should not contribute directly to the project’s core development.
Code of Conduct:
Participants are expected to maintain a respectful and inclusive environment. Any form of discrimination, harassment, or unethical behavior will result in immediate disqualification.
Projects will be evaluated on:
- Innovation: How original and creative is the project?
- Feasibility: Can the project be implemented or scaled in real-world applications?
- Impact: What is the potential influence of the project on its target domain?
- Presentation: How well is the project communicated through the poster and oral presentation?
The competition will celebrate outstanding contributions through recognition and exposure within the academic community.
Top Awards:
- Featured Publication on the UH-HPE Data Science Institute (DSI) website, showcasing the research and achievements of each winning team.
- Certificates of Achievement for all members of each winning team.
- Opportunity for winning teams to present their projects at future HPE DSI events.
Each winning team and its members will receive Top Awards, acknowledging their contributions to data science and AI research.
Number of Awarded Teams:
The number of winning teams will be determined and disclosed after registration closes, based on the number of participants in each category (undergraduate and graduate).
Modification of Rules and Decision Finality:
- The organizing committee reserves the right to modify rules or judging criteria as needed, with any changes communicated in advance.
- Decisions made by the judging panel are final and binding.
- Registration opens Saturday, March 1, 2025
- Registration closes Thursday, April 10, 2025
- Final presentation date Monday, April 28, 2025
Past Winners
First Place
Team: Saikiran Anugam, Department of Engineering Data Science, Hariharan Annadurai, Department of Chemical and Biomolecular Engineering
Project: Anugam and Annadurai investigated the relationship between chemical compositions and mechanical properties— such as tensile and yield strength— of industrial steels using machine learning and neural network models. Their research offers insights for optimizing manufacturing processes and improving material performance, with advanced models and robust error analysis enhancing the reliability of their findings.
Second Place
Team: Divija Kalluri, Computer Science Department(NSM) , Charan Gajjala Chenchu, Computer Science Department
Project: Kalluri and Chenchu proposed a method to improve X-ray interpretation accuracy using the DenseNet deep learning architecture. By combining segmented image features with diagnostic prompts and processed report data, they trained a large language model to generate concise medical reports. This system enhances radiologists' efficiency by automating image interpretation and report generation, providing a reliable tool for clinical diagnostics.
Third Place
Team: Rabimba Karanjai, Computer Science Department
Project: Karanjai’s AI-Powered Education Diary leverages advanced large language model technology to generate personalized code examples and study materials grounded in authoritative sources like textbooks and lecture notes. Continuous user feedback will refine the content, resulting in an intuitive, multi-device application that enhances personalized learning.
First Place
Team: William Le, Charles Tian
Project: Heart Disease Prediction using Deep learning
Second Place
Team: Khanh Nguyen, Osasenaga Obano, and Will Brieden
Project: Diabetic Retinopathy Classification Using Neural Networks
Third Place
Team: Wian van Dyk, Sammy Hamdi, Joshua Folh
Project: Brain Tumor Classification in MRI with Deep Learning
Fourth Place
Team: Jusvin Charles, Justin Jose, James David Graham
Project: Retinal Disease Detection Using Convolutional Neural Networks
Fifth Place
Team: Nirorn Hong, Erik Perales, and Maximus Furtado
Project: Age and Gender Prediction
First Place
Team: Tung Dinh, Sevban Sonmez and Benjamin Price
Project: Data Analyzation, Deep Learning and Cancer Diagnosis
Second Place
Team: Dosboi Allev, Kurmanbek Bazarov, Michael Moorman and Abraar Patel
Project: Detection of Lung Cancer Using Convolutional Neural Networks
Third Place
Team: Alex Burns, Isaac Burns, Allen Arani and Victor Artiles
Project: Detecting and Classifying Alzheimer’s Disease from MRI Scans Using Convolutional Neural Networks
Fourth Place
Team: Elliot Farmer Garcia and Clark Nelson
Project: Classifying Text Messages as Spam Using Convolutional Neural Networks
Fifth Place
Team: Nathan Acosta, Seyed Hosseini Darabi, Mohammad Mahmoudzadeh and Shaz Maknojia
Project: Using Convolutional Neural Networks [CNN] to Detect Invasive Ductal Carcinoma